AI, AI, AI… we keep hearing it everywhere. From groundbreaking innovations to everyday use apps, we’re flooded with new tech upgrades every day! And, all this becomes so overwhelming to keep pace with.
But it wouldn’t be frustrating if you know what you’re dealing with–the fundamentals, differences between key concepts, and the future of Artificial Intelligence.
We know that you’re here to understand artificial intelligence (AI), machine learning (ML), and deep learning (DL), in the simplest way possible. And, you’ve come to the right place.
These terms frequently dominate our discussions today and are often used interchangeably. But, all this becomes so confusing, and at the end of the day, you’re left wanting to have more clarity.
Well, that’s what we are going to do in this article. You’ll learn about:
- Differences between artificial Intelligence, machine learning, and deep learning—with clarity.
- Potential AI use cases
So without further ado, let's jump right in.
How are AI, ML, and DL related?
Before understanding the differences, let us take a step back and understand where it all started.
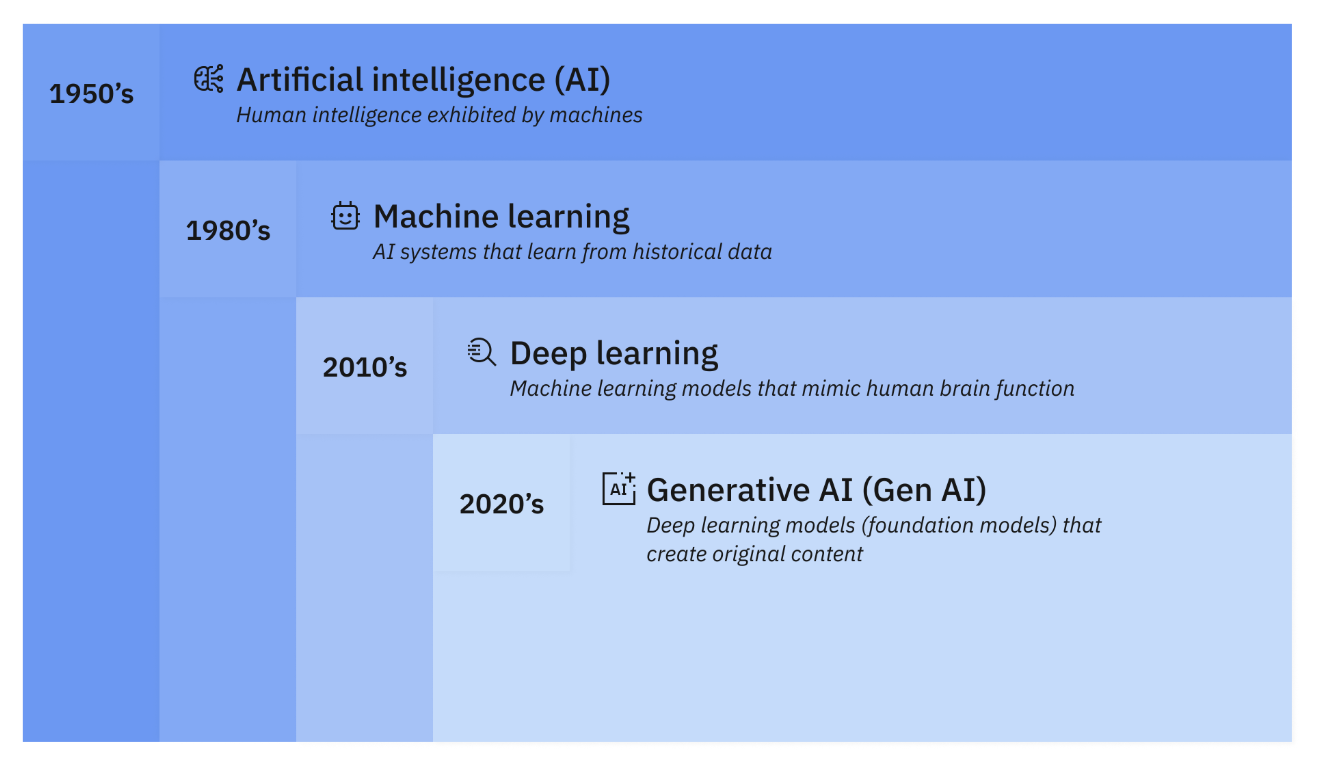
What is Artificial Intelligence (AI)
The term "artificial intelligence" was coined by John McCarthy in 1956 (yes, almost a century ago!), at the first AI conference held at Dartmouth College.
AI’s overarching goal is to create computers that can simulate or exceed human intelligence. It is a very broad field with different techniques aimed at enabling machines to learn, infer, reason, make decisions, and even show creativity.
Early AI efforts involved programming languages like Lisp and Prolog, which led to the development of expert systems in the 1980s and 90s. While AI adoption started slowly, recent advancements have dramatically increased its adoption in our lives and businesses.
What is Machine Learning (ML)
Machine learning is a subset of AI. Here, machines learn from data without being explicitly programmed for specific tasks. Instead of hard-coded rules, ML algorithms are trained on large datasets, allowing them to identify patterns, make predictions, and improve their performance over time.
Machine learning was more popularized around the 2010s and now forms the basis of many technologies we use. Several ML techniques exist today, including supervised, unsupervised, semi-supervised, and reinforcement learning.
Suggested Read: Machine Learning Guide
What is Deep Learning (DL)
Deep learning is a subset of machine learning that utilizes artificial neural networks with multiple layers (deep neural networks). They are used to analyze and process complex data.
These neural networks are inspired by the structure and function of the human brain, having interconnected nodes that process information in layers. The "deep" in deep learning refers to the presence of multiple hidden layers that help in getting insights from raw, unstructured data like text and images.
This reduces the need for human intervention. Deep learning has been instrumental in recent AI advancements, particularly in areas like natural language processing (NLP), computer vision, and speech recognition.
While classical ML often requires human intervention to label data and determine relevant features, deep learning can process unstructured data in its raw form and learn these features autonomously, making it highly scalable.
AI vs ML vs DL: A Quick Glance at the Differences
The Generative AI Revolution
The most recent advancement in the field of AI has happened in the field of generative AI. It is built upon deep learning and foundation models. It focuses on creating original content such as text, images, video, and audio in response to user prompts.
On a side note, some people have made the argument that generative AI isn't really generative. They think that it’s just regurgitating existing information and putting it in a different format. However, this argument doesn’t sit well with others.
For instance, in the case of music, every note has already been invented. So, in a sense, every song is just a recombination of some other permutation of all the notes that already exist. Composers are just putting them in a different order, and we don't say new music doesn't exist. People are still composing and creating new songs from the existing information.
Chatbots, virtual assistants, deepfakes, generating content and copy for marketing materials, etc. are the perfect examples of genAI.
What are Foundational Models?
These are deep learning models trained on huge amounts of raw, unlabeled data (like text from the internet) to serve as a base for various applications.
Large Language Models are a prime example, capable of predicting the next word, sentence, or even entire document with remarkable accuracy. Think of it as an advanced form of auto-complete but on a much grander scale.
The adaptability of these models is what makes them stand out. They've been in development for over a decade, becoming more sophisticated and complex along the way. This means they can handle a bunch of different tasks depending on what instructions they're given.
Think of them as a versatile tool that can be molded to fit many purposes! These models can also be used as a base for creating more specialized applications, opening up a world of possibilities.
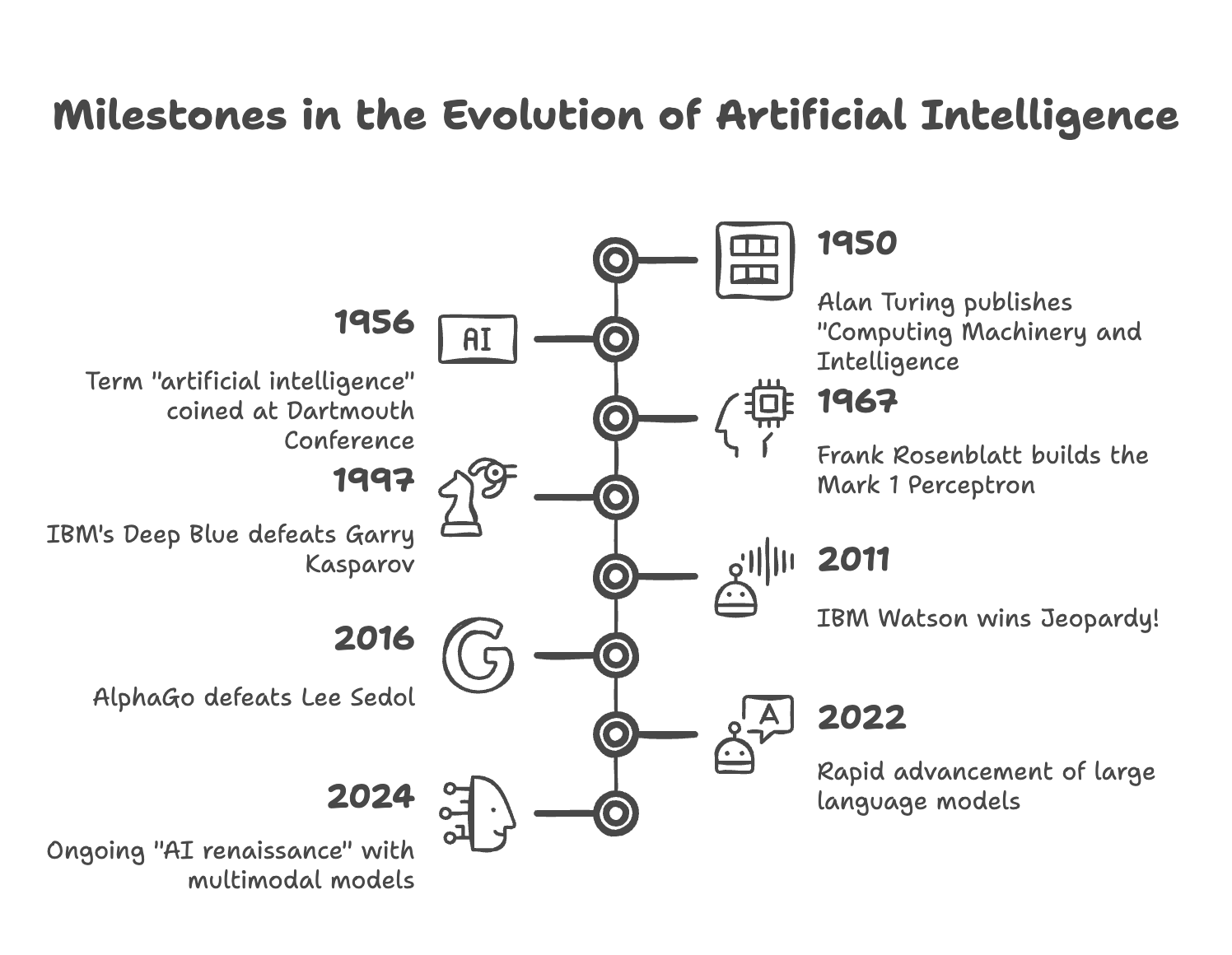
How Does AI Help Transform Enterprises (with use cases)
For enterprises, the implications of AI, ML, and deep learning are far-reaching. They offer opportunities to enhance their operations and offerings.
Automated Back Office Operations
GenAI can automate the boring stuff in logistics, like processing invoices, shipping manifests, and customs declarations. It can pull out the important info, sort things, and check for errors, which saves time and reduces mistakes.
It can also provide summaries of logistics reports, highlighting key performance indicators, bottlenecks, and areas for improvement. This can help your managers quickly understand the big picture without getting lost in the details.
GenAI is also amazing for financial automation. It can summarize financial reports and do a basic analysis to spot trends, anomalies, and potential risks. This gives financial analysts and managers a head start on their work.
When it comes to compliance and regulatory reporting, AI can automate the prep and submission of reports by grabbing and formatting data as needed. It can also keep an eye on compliance by analyzing documents and flagging potential issues.
Improved Customer Experience
AI-powered chatbots and virtual assistants can take care of customer questions, give quick answers to common questions, and pass on trickier problems to human agents.
This means customers can get help anytime, day or night, and get faster responses. These tools use NLP and generative AI to understand and respond to customer needs effectively.
Personalized support and recommendations can be given based on customer usage patterns and preferences, which are found through machine learning algorithms.
Smarter sales and marketing
AI is a game-changer for sales and marketing. It can help you create personalized marketing campaigns that really speak to each customer. By analyzing customer data, AI can figure out what products or services they're most likely to be interested in.
This means more sales and fewer customers leaving for your competitors. Plus, generative AI can even write personalized marketing copy for you!
AI can also help you score leads and figure out which ones are most likely to convert. This saves your sales team a ton of time and lets them focus on the hottest prospects.
With predictive analytics, you can even predict which customers are likely to churn, so you can take action to keep them on board.
Optimized Product Development
GenAI's ability to write code accelerates the new product development and simplifies the process of updating existing systems. It helps in the modernization of legacy systems and speeds up product development.
Additionally, predictive maintenance uses data analysis to anticipate and address potential issues within SaaS infrastructure, thereby preventing service disruptions and ensuring uninterrupted product availability.
Moreover, machine learning algorithms play a crucial role in SaaS cybersecurity by identifying any unusual or fraudulent activity. This enables the timely detection and mitigation of threats and unauthorized access attempts, safeguarding both the product and user data.
Future of AI: Why it Matters to Your Bottom Line?
Adopting AI, ML, and deep learning is truly beneficial for your enterprise:
- Saves time and effort by automating boring tasks, giving employees more time for creative and strategic work.
- Quickly analyzes tons of data to help make informed decisions based on facts.
- Boosts productivity, accuracy, and dependability in decision-making across all areas of the company.
- Minimizes human errors, like typos or miscalculations, in various processes.
- Provides 24/7 support and consistent performance through AI tools like chatbots.
- Creates personalized experiences that keep customers happy and coming back for more.
- Improves overall efficiency and cuts costs by streamlining processes and reducing waste.
The use cases that you saw earlier translate into saved time and money for the business—directly influencing revenue.
Many business leaders believe strongly in AI. They think the use of AI boosts productivity by at least 52%. They plan to invest even more in the time to come. We would see way more businesses using AI soon, which means AI is going mainstream.
This is happening because there is more data availability, better algorithms, and more high-end and powerful computers. Basically, AI is becoming a big deal in the business world.
Other future trends include the development of multimodal models that can process various types of data, leading to richer and more robust experiences. There is also a focus on creating small language models. These can be more efficiently trained and can achieve significant performance at a lower cost.
Current AI is mostly considered ‘weak’ or ‘narrow AI’. It’s designed for specific tasks, but the possibility of ‘strong AI’ is quite real. ‘Artificial general intelligence (AGI)’ would possess human-level intelligence across a wide range of tasks, but it remains a theoretical concept as of now.
The future of AI is exciting!
Closing Thoughts
AI adoption was once slow—held back by limited awareness and the belief that its real-world impact was still years away. But that’s changed. In just the last few years, rapid advancements have made AI not only accessible, but essential for forward-thinking enterprises.
As technologies like machine learning and deep learning have matured, they’ve moved beyond buzzwords to deliver real value—especially in complex business environments where precision, scale, and adaptability are non-negotiable.
But turning that potential into tangible outcomes still requires the right approach. That’s where Zams steps in.
From streamlining workflows to surfacing actionable insights and eliminating repetitive tasks, Zams helps teams do more—with less friction.
If you’ve been wondering how AI can actually move the needle in your organization, this could be a great place to start. Book a demo with us to see how Zam's agentic AI platform handles youe nuanced business operations.