Are you thinking about investing in agentic AI but unsure if it can handle the nuances of your business operations? You’re not alone.
Many enterprise leaders wonder if AI can truly adapt to their unique challenges.
The answer? Absolutely!
Just like autonomous vehicles learn and improve with every trip, agentic AI continuously adapts to your business environment, making smarter decisions over time. Agentic AI can help enhance financial risk management by making autonomous decisions based on real-time data analysis, thereby improving investment strategies and client outcomes.
In this blog, we’ll discuss real-world use cases where agentic AI has transformed operations, saving enterprises millions in costs and thousands of hours in manual work.
Sounds good? Let’s go!
Quick glance: Evolution of Artificial Intelligence (AI)
Artificial intelligence (AI) typically refers to the development of computer systems that can perform tasks typically requiring human intelligence, such as learning, problem-solving, and decision-making.
As AI systems have evolved over the years, it is now categorized into different types, including traditional AI, generative AI, and agentic AI.
Traditional AI focuses on executing pre-defined tasks based on rules or training data, often requiring significant human intervention.
Generative AI, on the other hand, excels in creating new content, such as text, images, and code, but still relies on human guidance for context and objectives.
Agentic AI stands out by enabling systems to make decisions and take actions independently with minimal human intervention. These AI agents can automate complex tasks, analyze data, and provide customer support, all while continuously learning and adapting from their interactions.
It’s worth noting that the research by Market US in 2024 shows that the US Agentic AI Market alone was valued at $.58 billion in 2024, with a robust CAGR of 43.6% and is expected to reach $58.91 billion by 2034.
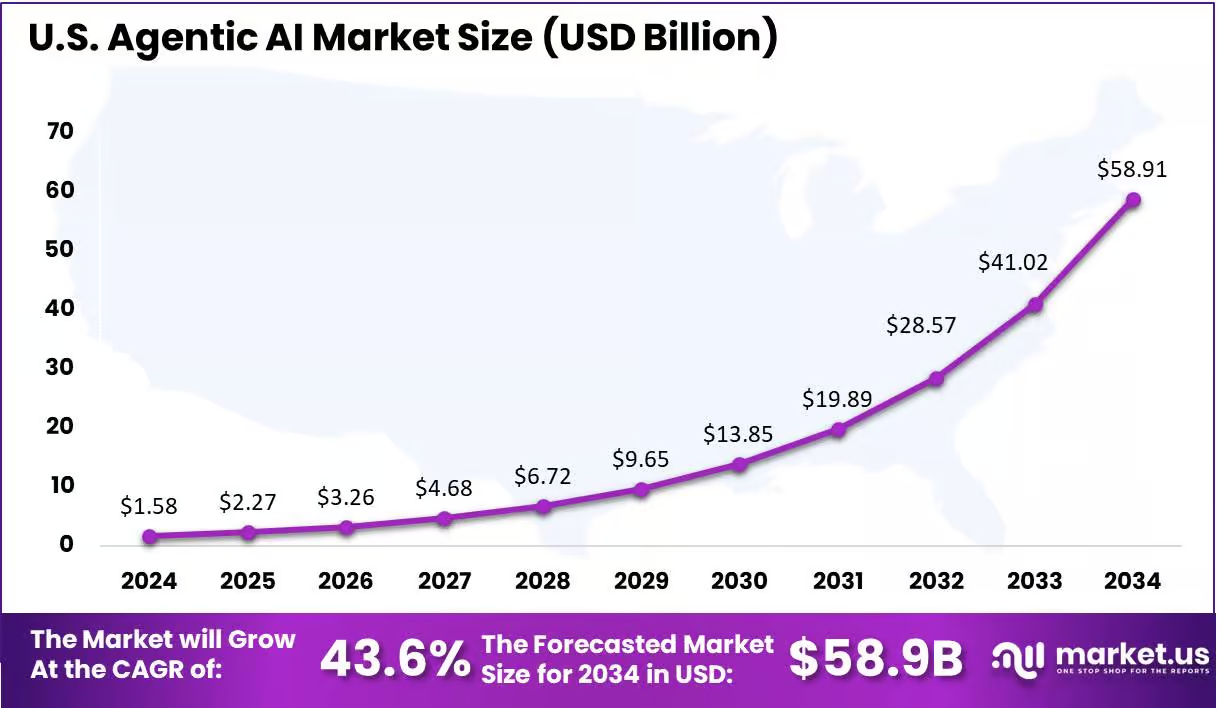
The development of large language models (LLMs) and advanced machine learning algorithms has accelerated the evolution of agentic AI, allowing these systems to understand natural language, reason, and learn from experience.
This makes agentic AI a powerful tool for businesses looking to enhance their operations and decision-making processes.
What exactly is agentic AI?Â
It all began with the first-generation chatbots, designed to simulate conversation through basic pattern recognition and decision trees. These rudimentary digital assistants laid the initial groundwork for what we now know as advanced conversational AI systems.
With the advent of deep learning, AI systems could understand context and semantics better, improving their ability to perform complex tasks and adapt to user interactions dynamically.
This leap in technology ultimately paved the way for agentic AI—a more advanced form of AI capable of independent reasoning, learning from interactions, and making decisions without human intervention. Agentic AI systems can now understand and process nuanced language, offer personalized responses, and handle intricate queries, reflecting a significant evolution from their chatbot predecessors.
Agentic AI differs from traditional AI primarily based on its ability to operate autonomously, whereas traditional AI is more focused on executing pre-defined tasks based on rules or training data.Â
Unlike traditional AI models where frequent human intervention is needed, agentic AI analyzes the tasks at hand and makes decisions with minimal input.
Think of it as an autonomous entity that perceives its environment, collects data, makes decisions, takes action and even learns on its own to achieve specific goals.
It’s like having a virtual assistant that gets the hang of what you need, based on your business context, figures out a plan and takes specific actions—handling tasks and complex workflows pretty much on its own.
Key Features of an agentic system
Agentic AI boasts several key features that set it apart from traditional AI and generative AI. One of its most significant attributes is the ability to make decisions and take actions independently, reducing the need for constant human oversight.Â
This autonomy is powered by advanced natural language understanding, allowing agentic AI systems to comprehend and respond to complex queries in a human-like manner.
Another standout feature is the capability to learn from experience. Agentic AI systems continuously analyze data, identify patterns, and refine their decision-making processes over time. This adaptability ensures that the AI remains effective even as business environments and data inputs change. Additionally, agentic AI can automate repetitive tasks, streamline complex business processes, and provide valuable insights to support decision-making.
Agentic AI tools can be seamlessly integrated with enterprise data and existing systems, enabling organizations to implement solutions that drive significant business value. The key differences between agentic AI and other types of AI lie in its ability to operate with minimal human intervention, handle complex scenarios, and make decisions based on real-time data.Â
This makes agentic AI an invaluable asset for businesses looking to enhance efficiency and responsiveness.
How does Agentic AI compare to Generative AI?
When you compare agentic AI with generative AI, it becomes clear that they serve distinct yet complementary roles.
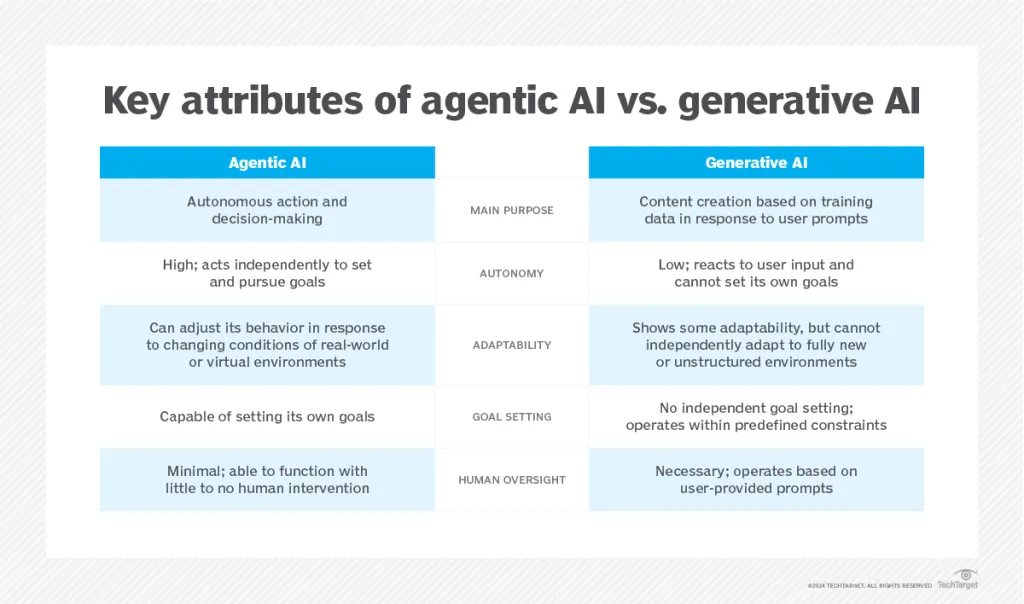
Generative AI: The creative powerhouse
Generative AI is all about creation. It has the capability to produce new and original content across multiple formats, including:
- Text: Crafting essays, articles, and marketing copy.
- Visuals: Generating images or videos from simple prompts.
- Audio: Composing music or synthesizing speech.
- Code: Writing snippets or entire programs.
It typically draws from existing data to generate ideas and solutions. It's fundamentally focused on content production where human guidance is often necessary and recommended to define the objective, context, and edits.
Agentic AI: The autonomous executor
In contrast, agentic AI is autonomous and action-driven. It is engineered to support systems that make independent decisions and take actions without requiring constant human oversight. It excels in:
- Analyzing complex circumstances.
- Devising strategic plans and methods.
- Executing tasks autonomously based on predetermined goals.
It can adapt to dynamic environments and learn from its interactions, effectively functioning without the need for detailed input once it’s set up. Agentic AI takes autonomous functions to the next level, operating independently of human input and utilizing advanced technologies like large language models and machine learning.
While generative AI thrives on creativity, agentic AI shines in application and execution. By harnessing both, you can create comprehensive solutions. For example, generative AI can develop engaging marketing materials, and agentic AI can efficiently distribute these materials across various platforms, optimizing for performance in real-time.
Together, they bridge the gap between idea generation and practical implementation, offering a full-spectrum solution to complex challenges.
Agentic AI operates in four key stages:
- Perception (Gathers data from the world around it)
- Reasoning (Processes the gathered data to understand the context)
- Action (Decides and takes action based on its understanding)
- Learning (Continuously improves and adapts over time—based on feedback and experience)
Now, let’s look at some real-world examples of how these AI agents typically automate tasks, provide real-time insights, enhance decision-making processes, and more.
To keep it real, verifiable and earn your trust, let me take you through use cases of some of our customers — which involves solving for some of the most complex and nuanced business operations.
Agentic AI use cases
1. Agentic fee prediction to prevent revenue leakage upwards of $1 million
One of our customers from the logistics industry was facing challenges in managing unanticipated accessorial fees, which tends to be a common occurrence during shipping operations. As you can imagine, unanticipated fees disrupt shipment planning and strain carrier relationships.
The impact wasn’t just on operations but also on business consequences as the unanticipated fees led to financial losses. However, due to lack of insights these unexpected expenses were hard to mitigate.
Solution? An agentic AI solution with a nuanced predictive classification model.
A model that perceives the environment in which the logistics company operates, reasons by analyzing a range of input variables, understands the context, anticipates accessorial fees and provides real-time predictions seamlessly within their existing logistics management platform.Â
By effectively processing and interpreting large volumes of financial data, the agentic AI solution improved investment strategies and operational efficiency.

The proactive identification of potential fees resulted in significant annual savings exceeding $1 million. Our 70% accuracy rate in predicting fees significantly outperformed the industry average of 50%, which substantially reduced revenue leakage.
2. Agentic invoice extraction to save 4160 hours of manual labor
One of our customers, who manufactures industrial hardware, were manually managing 100s of invoices on a weekly basis, leading to delays, data discrepancies, and inefficiencies impacting financial workflows. As you would expect, this led to key stakeholders spending their valuable time on repetitive administrative tasks.
Zams solution? Agentic invoice extraction that processed and extracted key information from invoices including vendor names, dates, amounts and payment terms. By integrating the agent into their account and ERP system, they not only ensured a smooth workflow but also continuous learning and adaption, thus ensuring high accuracy and scalability.Â
The system can autonomously execute tasks such as processing and extracting key information from invoices, significantly reducing manual effort.

The automated processing using the agentic invoice extraction saved at least 10 hours per week per team member saving 4160 hours annually otherwise spent on manual data entry. The invoice processing time came down to 30 seconds per invoice, and the error rates dropped by 98.5%
3. Agentic employee churn prediction leading to $1.9 million in savings
Another customer who is a customer support service provider was experiencing an annual employee churn of over 35% where approximately 8,750 agents were leaving the company every year.
High churn rate meant recruitment, training and operational disruption cost approximately $15,000 per agent, leading to loss in millions of dollars per year.
The solution? A predictive churn model that embedded into the existing systems to understand the reasons for previously churned employees, also continuously learn about the change in context. Then it compares the learnings with new candidates and employees and assigns risk scores helping recruiters to prioritize the right candidates.Â
By automating repetitive tasks and streamlining core HR operations, agentic AI solutions can significantly enhance human resources management.

As of now, the churn rate has come down by 15% saving $1.69 million in revenue within the first year. Given that the model continuously adapts to the changing workforce dynamics, the effectiveness and reliability of predictions and risk scores will not only remain high but continue to improve.
4. Agentic forecasting of energy consumption adding $775K revenue
One of our customers who is a provider of renewable energy solutions had an interesting scalability problem they were trying to solve. Inaccurate forecasting of energy consumption across their grids in multiple geographic locations was leading to revenue losses, inefficient distribution and missed opportunities in scaling.Â
It also directly impacted their ability to effectively optimize grid pricing, and negotiate favorable energy contracts.
Solution? Agentic multiple time series forecasting! Our models connected to their live energy usage database, leveraged historical energy usage data to provide actionable insights into consumption patterns.
The models continuously learn and adapt to changing energy consumption patterns including grid-specific consumption trends, weather patterns, regional demand fluctuations, and more.

The accuracy and reliability of the models helped the company optimize grid pricing, distribute resources optimally, negotiate better contracts, and meet demands efficiently resulting in $775K in additional revenue, within the first year.
5. Agentic credit review attributing to $625K in annual cost savings
Here’s another interesting story. Another customer who provides venture debt solutions to growth-stage companies, faced an interesting challenge. Their lawyers review lengthy documents such as Shareholder agreements, Articles of Incorporation, Capitalization Tables, and more to assess the creditworthiness of potential clients.
The time consuming manual process was leading to delays in funding decisions and onboarding new clients. In short, it was high operational cost and slow customer acquisition.
Solution to save cost and time? Agentic credit review model tailored to analyze critical legal documents where the solution extracts and summarizes key details such as ownership structures, voting rights, and financial obligations.
Since these models self-learn and adapt to variations in documentation structure, they ensure long term reliability and scalability as new document types and formats emerge. The agentic AI system can handle complex processes such as analyzing critical legal documents and summarizing key details, significantly reducing operational costs.

At an average lawyer billing rate of $500/hour, the automated solution saves 1250 lawyer hours annually, assuming an average of 5 hours per document and 250 documents per year. The time saved translates to $625K in annual cost savings, significantly reducing operating costs.
AI powered customer engagement
AI-powered customer engagement leverages advanced AI systems to interact with customers, provide support, and personalize their experiences. Agentic AI can significantly enhance customer support teams by automating routine tasks, analyzing customer data, and delivering personalized responses to customer queries. This not only improves efficiency but also ensures that customers receive timely and relevant assistance.
For sales teams, AI-powered agents can analyze market trends, identify potential customers, and offer personalized recommendations, thereby driving sales and improving customer satisfaction. By streamlining customer engagement processes, agentic AI helps organizations reduce costs and enhance the overall customer experience.
With agentic AI, businesses can provide instant access to support, personalize customer interactions based on past interactions and preferences, and ultimately drive business growth. The ability to automate routine tasks and provide intelligent, context-aware responses makes agentic AI a game-changer in customer engagement.
Technical aspects
From a technical perspective, agentic AI is powered by sophisticated machine learning algorithms, large language models, and natural language processing (NLP) techniques. These components enable agentic AI systems to understand and generate human-like responses, making them highly effective in various applications.Â
Integration with external tools and existing systems allows organizations to leverage their current infrastructure and data, maximizing the benefits of agentic AI.
Developing an agent system requires expertise in AI, machine learning, and software development, as well as a deep understanding of business operations and customer needs. While every team needs to be powered by agentic AI — not everyone has the expertise, and even if you do, the question is — is that the best use of your time?
That’s where a platform like Zams and it’s done for you teams make a massive difference.

With Agentic AI you can write code, generate software, and automate repetitive tasks, freeing up human employees to focus on more complex challenges. This not only drives operational efficiency but also improves decision-making and reduces costs.
Implementing agentic AI involves careful consideration of its unique capabilities, such as handling complex scenarios, reviewing code, and providing human judgment when necessary.Â
By leveraging agentic AI, you can achieve significant improvements in operational efficiency, decision-making, and customer satisfaction, making it a valuable addition to any business strategy.
The future in terms of automation
Today’s Agentic AI and automation is a big leap from the AI we know so far. Fast growing organizations and those with ears to the ground integrate these technologies, leading to streamlined operations, collaboration and decision-making.
Agentic AI is not just about doing single tasks; it understands its environment, makes decisions, and works towards your goals. Â
The progress we see today is just the beginning. In the next 2-3 years, we expect to see a fundamental shift in how companies operate. Automation and AI will permeate all levels of the business, leading to unprecedented efficiency and productivity.Â
The future of AI applications includes more natural conversations, creative content generation, and sophisticated decision-making processes, enhancing user interactions and improving outcomes in various fields.
Most importantly, it’s not just for techies—anyone can use it to automate tasks, understand data, and make things better for customers and employees.
Companies will differentiate themselves through superior customer engagement, agility in adapting to change, and enhanced employee engagement, thanks to AI-driven insights and workflows.
In essence, the future of agentic AI and automation holds endless possibilities, promising a world where human and machine collaboration leads to unparalleled advancements and efficiencies.
Want to see how your enterprise can use agentic AI? Book a demo with Zams today!